Research Interests
MD of Soft Matter
Soft materials are highly correlated many body systems with complex structures and dynamics spanning a wide range of length and time scales. Their relaxation processes involve complex phenomena such as vitrification, jamming, entanglement and semi-crystallization, which are highly process-dependent and for which no comprehensive theoretical framework and understanding exist. Optimal use of soft materials in future electronics, medicine and energy devices demand a deeper understand of these phenomena. My objectives are to improve the fundamental understanding of these phenomena in polymer and other soft matter systems, and establish their design rules for engineering applications.
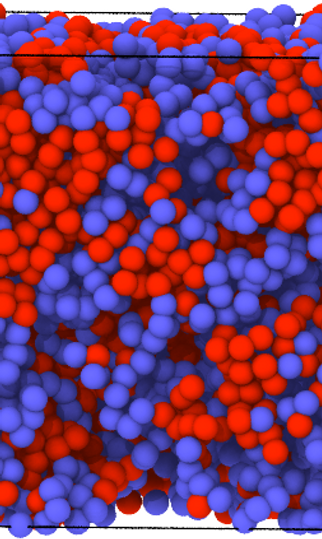
Deep Neural Network Models for Soft Materials
A central barrier in improving the fundamental understanding and design of soft materials is the unavailability of transferable potential models that accurately describe inter-particle interactions in soft matter systems for a wide range of physicochemical conditions. The current fixed functional form based approaches pose limitation on the physics and chemistry that can be captured. On the other hand, fully atomistic models are limited by their short time and length scales. To address these limitations, I develop fully flexible coarse-grained deep neural network potentials that capture polymer dynamics accurately, and conduct large-scale molecular simulations for predicting macroscopic properties.
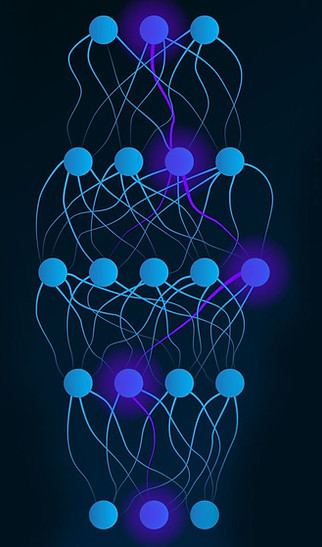
Publications
1. Entropic Cohesion in Vitrimers
R Karmakar, Himanshu, S Sastry, SK Kumar, TK Patra
arXiv preprint arXiv:2402.16226
Under Review in ACS Macro Letters
​
2. Extrapolation of ML Models for Predicting Extremal Properties of Copolymers
IH Hashmi, Himanshu, R Karmakar, TK Patra
Under Review in Molecular Systems Design & Engineering
​​
3. Developing efficient deep learning model for predicting copolymer properties
Himanshu, K Chakraborty, TK Patra
Physical Chemistry Chemical Physics 25 (37), 25166-25176
​​
Himanshu, TK Patra
arXiv preprint arXiv:2210.06622
​
5. Book Chapter on "Plastic Waste Assessment" in "Global Waste Management"
A Ahamad, Himanshu, B Kumar, P Kumar
Scrivener Publishing, Wiley.
(Proofread sent)
Conferences
1. COMPFLU-2023: Role of dynamic crosslinking on the miscibility of polymer blends.​
Himanshu; Karmakar, R.; Kumar, S.; Patra, T.
(Poster)
​
2. APS March Meet-2023: Developing Universal Machine Learning Model for Predicting Polymer Properties.
Himanshu; Patra, T.
(Oral Talk)
​
3. COMPFLU-2022: Deep Learning Models to Predict Sequence-Defined Properties of Polymers.
Himanshu; Patra, T.
(Poster)
​
4. SCHEMCON-2020: Impact of lockdown and the trends after unlock on air quality of Delhi, Chennai and Mumbai during COVID-19.
Himanshu.
(Oral Talk)
​
5. ACES-2020: Nanofluids- History, Recent Development, and Various Applications.
Himanshu; Choudhary, P.; Pandey, A.
(Poster)